The value of predictive maintenance comes not only from replacing old technology with new technology. The powerful combination of algorithms and the human-computer symbiosis can take your maintenance to a higher level.
Algorithms and processes
The second industrial revolution, which mainly took place in America and revolved around the replacement of steam engines with electricity, did not bring about any productivity gains in the first few decades. The old technology (steam engines) was simply replaced with new technology (electricity). It was not until a new generation of managers took over that productivity was boosted by taking advantage of the flexibility of the new technology and adapting processes accordingly. This condition also applies to predictive maintenance: the real economic value will only be unlocked if structural process adjustments are also made.
A good example of such a process adjustment is the shift from a push to a pull principle. This massively increases the efficiency and effectiveness of maintenance. Maintenance is traditionally organized according to a push principle. A maintenance schedule is drawn up based on the supplier's data, and maintenance is carried out at fixed intervals. These schedules are based on a pessimistic approach, taking into account poorer performers in the group and a highest common denominator, and therefore not the state of an individual machine. In providing insights into the current state and making predictions about the future state of a machine, we can also make the shift to a pull principle for maintenance activities. The pull principle is based on the 'customer's' demand and only produces amounts in accordance with this demand. The future state of a machine will then trigger maintenance activities, thus creating the demand for maintenance. Demand is initiated by a predictive maintenance algorithm, but this will only lead to an effective maintenance demand in combination with the engineer's expertise.
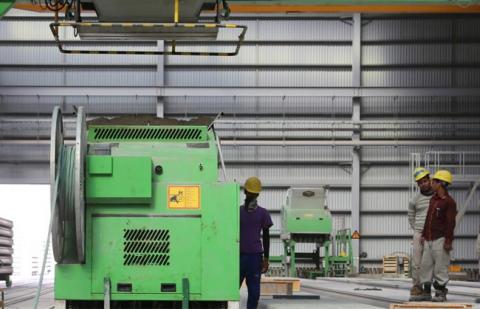
Human-computer symbiosis
A machine has not turned into a database and data is not sacred. A machine is still primarily a matter of mechanics. The combination of the data-based insights and the engineer's expertise is therefore the second key to real economic value. A machine that is being converted to make new parts produces "new" data, and the algorithms will have to be adapted accordingly. In an initial phase, the margin of error for such a machine is greater and the interpretation must take this into account. In addition, it is self-evident that situations that have not yet occurred cannot be predicted on the basis of historical data.
Historical data in itself provides a predictive maintenance paradox. The preventive maintenance that is carried out helps to prevent corrective maintenance activities as far as possible. This implies that the historical data only includes the problems that could not be avoided by preventive maintenance activities, i.e. the exceptions. This provides companies with highly biased data: data that is not suitable for developing predictive maintenance. On the other hand, companies often have no or only a limited amount of historical data. In these cases, unsupervised learning algorithms offer a potential solution.
Unsupervised learning algorithms rely on train models using baseline data rather than labeled data to define normal machine behavior. New data from sensors is evaluated against this baseline data, which characterizes a behavior and maps out any deviations from normal behavior. Vibration is a popular and proven effective sensor value. It is possible to identify deviating behavior very accurately in an automatic way by measuring the vibrations on a machine component. However, we have not reached this point yet because vibrations are a symptom of an underlying problem. This brings us back to the maintenance engineer who has to use the symptoms to determine the real cause. Engineers who examine the differences in sensor values such as vibration, power consumption, temperature or pressure, will be able to perform a thorough analysis and request a maintenance interval in a more accurate way. This is a perfect application of the pull principle.
We are able to evaluate the impact of the maintenance activities by actively monitoring the machine's sensor values. Does the machine turn back according to the baseline after maintenance or do we continue to notice deviating behavior and is there still a risk of a possible breakdown? Improvements can also be made here by continuously evaluating the maintenance activities.
A positive impact in many areas
Switching from a push process principle to a pull principle for maintenance is done in a very gradual way. The maintenance intervals gradually shift based on predictions that are reinforced by the knowledge and experience of employees. Step by step, the amount of maintenance is reduced to an optimal pointand a cost reduction is achieved. The economic value is not only limited to a reduction in maintenance costs; there is also a positive impact on the quality, safety, lifetime and uptime of the machines. The latter – the uptime – has a direct link to turnover. Every improvement in uptime results in an increase in production, and this illustrates the high economic value of predictive maintenance. Predictive maintenance will not only shift the maintenance intervals over time, but will also advance maintenance activities as necessary in order to avoid downtime. A PwC research study shows an average uptime improvement of 9% as a result of switching to predictive maintenance. You can start calculating.